Visualizing
the Virus
Modelling the Virus
Tracing the fault-lines of Epistemic Justice in Disease Modelling and Data Visuals
It’s rare to hear politicians so bluntly reveal the biopolitical calculus behind public health policies as has been the case with Boris Johnson. Framed in the populist rhetoric of ‘Freedom Day’, Johnson announced an end to all COVID-related restrictions from July 19 onwards. No more wearing of masks or social distancing rules in private and public spaces, and unlimited and unchecked access to all social venues, including nightclubs and sport stadiums. At least in England. Sadly, the prime minister said, there will be more deaths but we have to balance the risks of the disease, including the loss of life and prolonged suffering, with the risks of legally enforced restrictions that take their own toll on people’s lives and livelihoods.
Johnson’s decision has been met with fierce criticism from scientists, public health officials, and concerned members of the public. Lifting all public health measures in spite of rising infections was perceived as ‘reckless’, ‘a dangerous experiment’ or ‘epidemiological stupidity’ by the WHO and national health experts. The number of cases, scientists warned, are likely to rise to three times the current rate, reaching an estimated 200,000 per day, if all protective measures are stopped. The number of long COVID cases will also go up in their thousands as a result of the policy change. Putting an end to all restrictions at this point risks burdening an entire generation with long-term health.
Boris Johnson was not unaware of the worrying figures emerging from the scientists’ epidemiological models., yet he was determined to go ahead anyway. Modelling disease spread shows that the number of COVID-related deaths is falling, which led him to conclude that, having vaccinated 52% of the population helped to greatly reduce the mortality rate, it’s now up to herd immunity to do the rest.
The controversy over Johnson’s COVID policy points to peculiar tensions at the heart of scientific modelling, in particular with regards to governmental decisions in the name of public health or the common good. The fact that two radically opposed recommendations could be derived from one and the same data set calls for a closer look at computational models as epistemic objects, in the light of knowing that they can be mobilized to legitimate fundamentally contradictory public health strategies.
Epidemiological models that simulate how and where viruses spread have proven an indispensable tool for governments in the monitoring of infections and the identification of local clusters and risk zones. Being able to visualize and compare the effectiveness of public health measures such as lockdowns, school closures or the occupancy of intensive care units and hospital beds provides a powerful instrument, in particular in situations where conclusive evidence about hard or soft lockdowns, wearing masks and the scope of protection of vaccination is lacking, leaving plenty of room for very different judgements on what can, or should be done to protect and control the situation and to prevent premature deaths. And yet the irregularity and inconsistency of empirical evidence and the divergence of conclusions capable of being drawn from simulating possible scenarios also exposes critical epistemological fault-lines in the ways computational models are used to confront scientific uncertainty and inconclusiveness. As Taylor rightly notes, this illustrates how data visualizations can be used by politicians ‘to perform public clarity of purpose’ without necessarily understanding what the best course of action is.
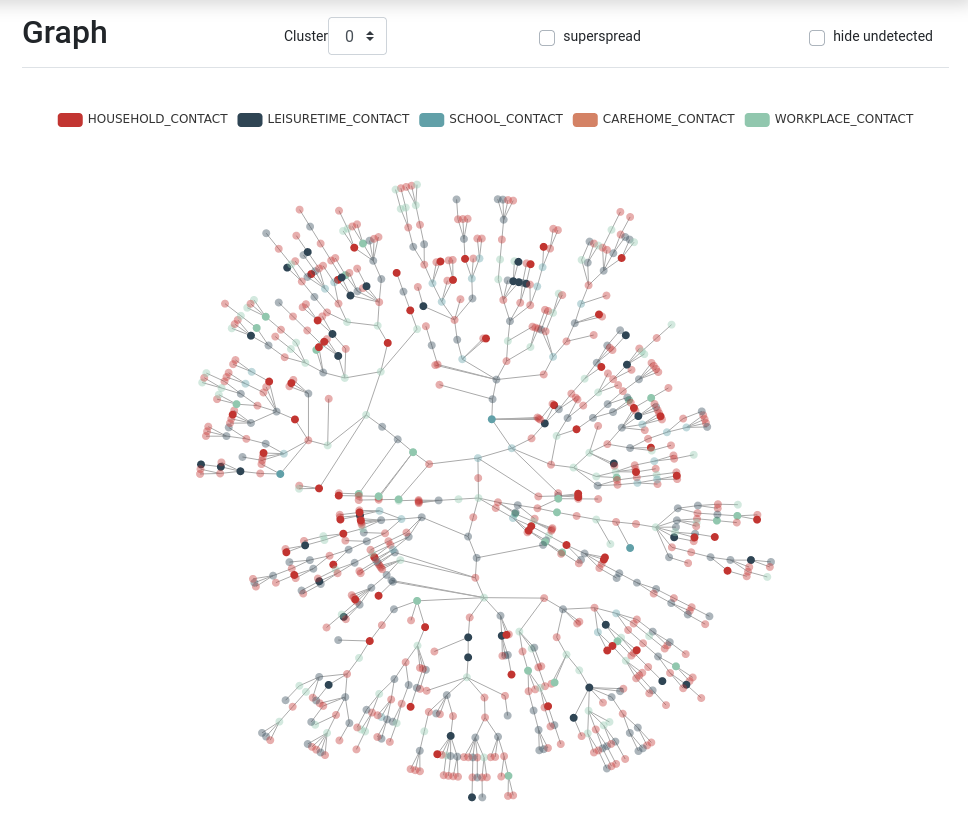
Computing infection paths ways in a Viennese District, Spring 2021 © dwh simulation services, Vienna, Austria
This ability ‘to perform public clarity of purpose’ without a comprehensive grasp of a situation begets the question where to situate the knowledge generated by visual epidemiological models and how it articulates principles of transparency, accountability and contestation in processes of decision-making that affect the well-being, health and livelihood of a population in democratic governance.
At their core, simulations can be described as mathematical models that replicate and explore complexities in digital objects or visual form, i.e. networks, graphs, vector-based maps etc. They are neither plain theory nor sole experiment, but oscillate somewhere between speculative fictions, empirical observation and dynamic renderings of system processes that imitate or construct the situations they seek to understand. A widely used method for such evaluations are so-called agent-based models. These combine key socio-demographic data, mobility patterns, population density and infrastructural arrangements with medical statistics to explore how infections are passed between individuals in the course of daily routines, such as going to work or school, or engaging in some leisure activity. A key advantage of agent-based models over other forms of modelling is that they do not simply apply abstract formulas for measuring the exponential spread of the virus, but continuously adjust their basic assumptions and parameters to observed patterns of spread and infection rates. This does not mean that agent-based models are free from ideal-type generalizations and inferences, but they nonetheless provide a more nuanced and realistic basis for anticipating trends and for comparing possible effects of hard and soft lockdowns, school closures, night curfews, etc., than purely mathematical calculations of infection rates and disease spread.
And yet, even agent-based models are only as good as the data used to simulate possible outcomes and scenarios. The problem here is not only that the testing and contact-tracing mechanisms currently in place inevitably miss those who are unable or unwilling to undergo medical tests, whether because they lack access to the health system or are hesitant to use it for fear of compromising their legal status or losing their jobs. The problem is, that these models rely heavily on publicly available or governmental data – the spatial distribution of settled areas (villages, cities, towns), road and public transportation networks, hospital locations, business districts etc., – that implicitly assume regularized citizens, with a fixed address, work-place and commuting habits as a generalized template for mapping and evaluating social relations and behaviour with regards to risks of infection and disease spread. This is to say, epidemiological models are prone to reproduced existing inequalities and gaps in social welfare and healthcare provision, if they are not routinely scrutinized and challenged for absent variables – lack of data on vulnerable groups, such as undocumented workers, migrants, refugees and other marginalized populations that remain invisible, and hence insufficiently addressed. Such a reading of data models against the grain has widely been missing in the public debate of infection scenarios and policy responses, leaving plenty of space to mobilize what are inevitably simplified mathematical abstractions of social complexities, in order to justify generalized restrictions that ignore their highly uneven effects. In this way the critical tasks of saving lives by way of data visualization ended up hiding the differential effects of policy interventions on different segments of the population, while at the same time concealing structural weaknesses and discrimination – the irregularisation of certain bodies, professions or social activities – as a specific source of vulnerability and risk.
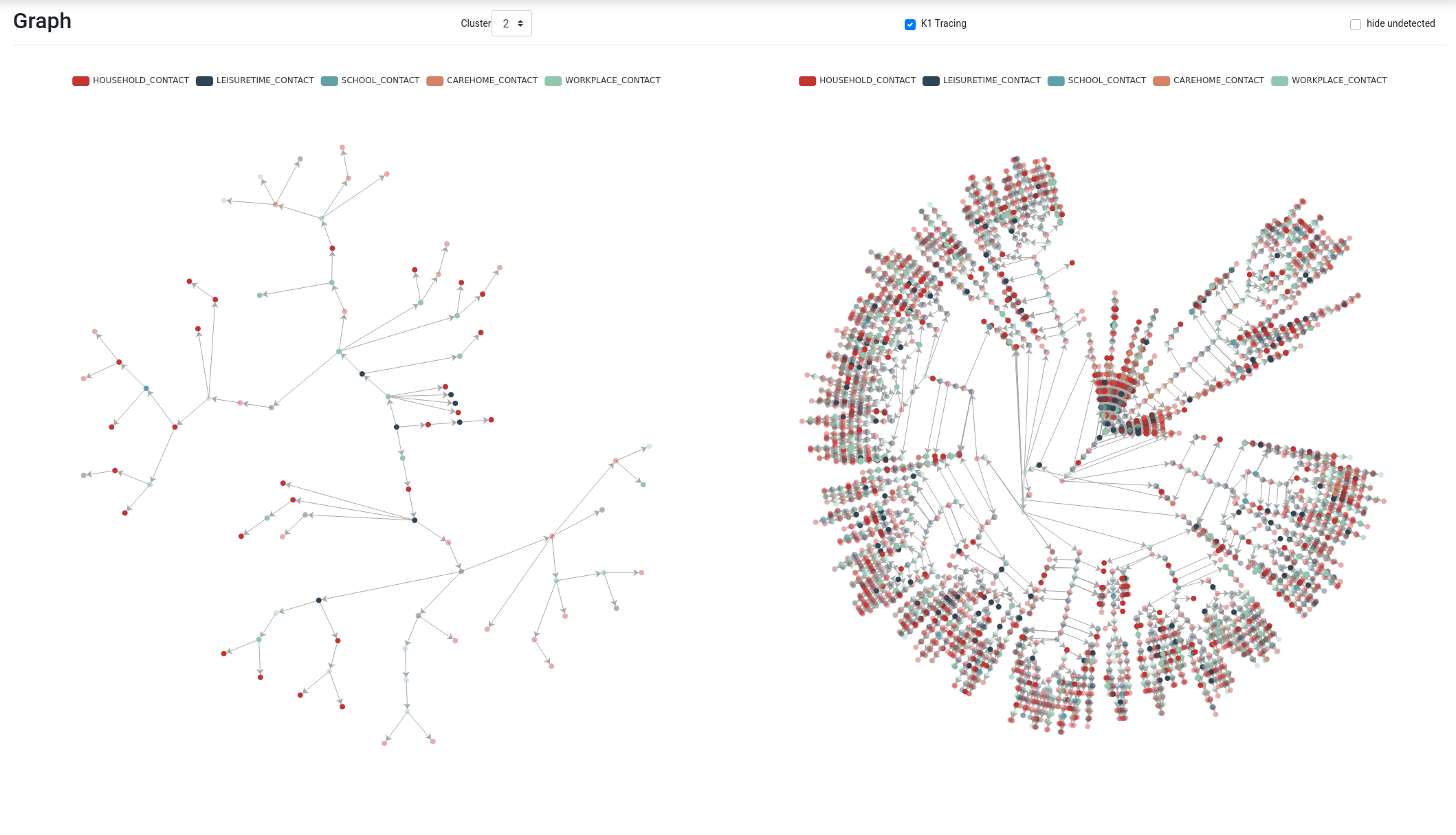
Dual Circular Graphic of Computing infection paths ways in a Viennese District, Spring 2021 © dwh simulation services, Vienna, Austria
Against this backdrop, Boris Johnson’s announcement of ‘Freedom Day’ appears dishearteningly honest. He openly admits that his government is ready to accept a threshold of COVID-related deaths for the sake of the well-being of the rest of the population – a confession that in other contexts is skilfully disguised in the generalized figure of available ICU units or hospital beds. To say that is not to condone Johnson’s policy of individualizing risk by outsourcing the responsibility for public health to individual behaviour and the force of nature – herd immunity. Highlighting this is intended to raise an altogether different question about the openness and transparency of scientific models to contestation, and to review the mechanisms in place for holding evidence-based policy decisions to account.
Epidemiological models never produce a readily available, transparent object or representation of societies. They are dynamic renderings of social systems in which particular ideas of risk, vulnerability, relevance and dependency of individuals, groups and institutions are enacted and made visible in distinct ways. Simulations of disease spread, in this sense, provide a powerful platform for engaging in collective thought experiments about the social visions and imaginaries underpinning public policy and healthcare systems so that they can be tested and opened to public scrutiny and debate. Yet this potential remains widely underutilized, not only in the UK.
What is needed is a careful review of the mechanisms and means in place for scrutinizing speculative policies derived from scientific models, to ensure that epidemiological models are subject to the same democratic scrutiny and review as other legal measures, and to reflect the full plurality of voices and standpoints in decision-making that a transparent, fair and socially just process of political deliberation demands. This would entail making sure that scientific models and data visualization reflect the full spectrum of system processes and relations that constitute the diversity of interests and concerns in a given population, irrespective of their legal status. But it also includes opening scientific models to questions from critical individuals and groups outside the closed circle of health experts, epidemiologists and public health officials, who have kept the critical task of defining the basic building blocks of data models and simulations firmly in their hands.
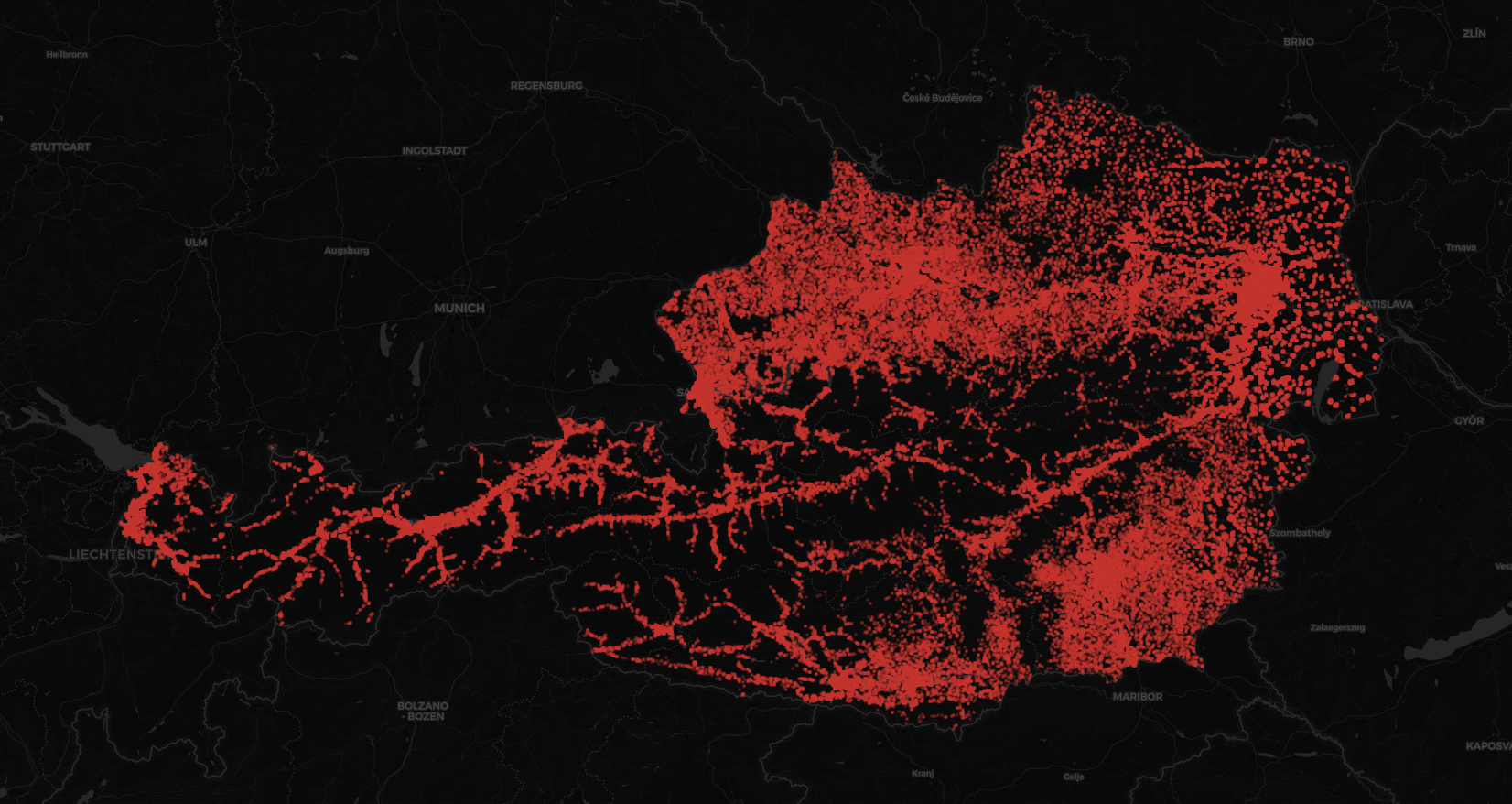
Simulating Disease Spread in Austria, Spring 2021 © dwh simulation services, Vienna, Austria